
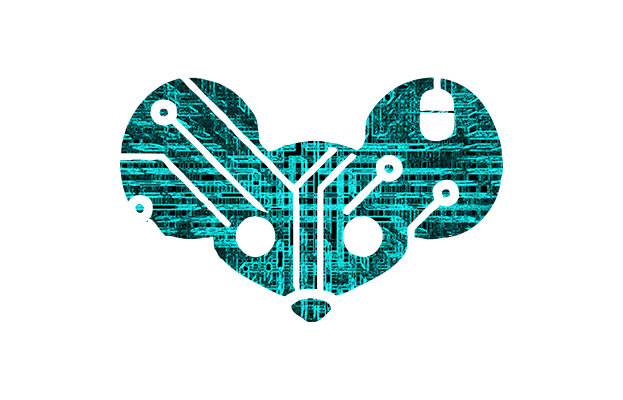
it’s super weird that people think LLMs are so fundamentally different from neural networks, the underlying technology. neural network architectures are constantly improving, and LLMs are just a product of a ton of research and an emergence after the discovery of the transformer architecture. what LLMs have shown us is that we’re definitely on the right track using neural networks to solve a wide range of problems classified as “AI”
i feel like this is a big part of it. it reminds me of the Sapir Whorf Hypothesis. search results and neural networks are susceptible to bias just like a human is; “garbage in garbage out” as they say.
the quote directly after mentions that newer or more precise searches produce more coherent results across languages. that reminds me of the time i got curious and looked up Marxism on Conservapedia. as you might expect, the high level descriptions of Marxism are highly critical and include a lot of bias, but interestingly once you dig down to concepts like historical materialism etc it gets harder to spin, since popular media narratives largely ignore those details and any “spin” would likely be blatant falsehood.
the author of the article seems to really want there to be a malicious conspiratorial effort to suppress information, and, while that may be true in some cases, it just doesn’t seem feasible at scale. this is good to call out, but i don’t think these people who concern their lives with the research and advancement of language concepts are sleeping on the fact that bias exists.